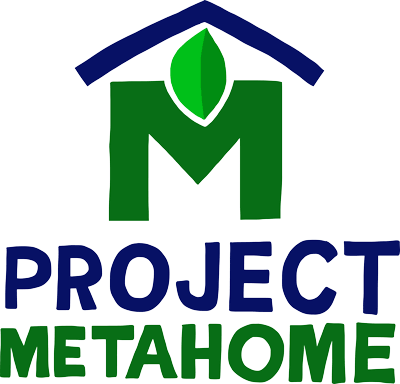
Common Sensors CrossTalk
Inside MetaHome, we use many sensors, and with many new communications networks emerging (LTE, 5G, etc.) as potential connectivity routes, that number expands exponentially. Sensor data in IoT is a wonderful thing, but what happens when that data, intended to feed a zone quality target, becomes corrupted? Sensor functionality cannot always be easily calibrated or checked.
Let’s examine a simple issue to illustrate the problem. MetaHome uses zones, each of which is given target quality standards for six different environmental characteristics.
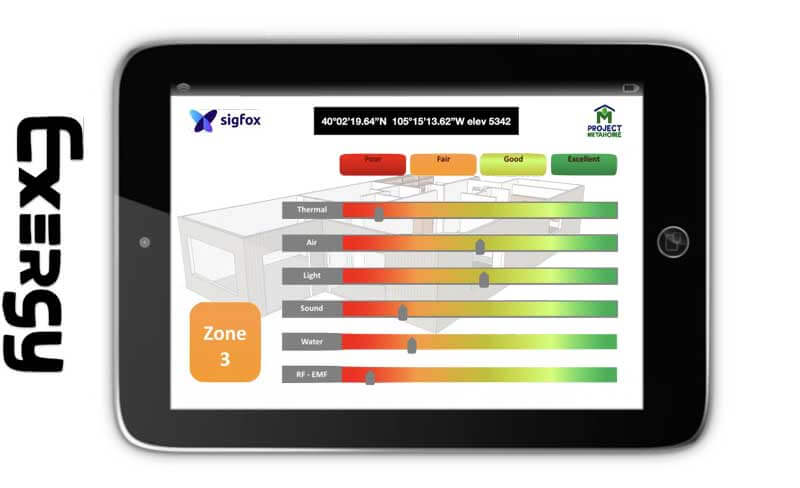
The sensor network reports whether the standards are being met in each zone, and if not, why. This is important because the system is tied to many service level agreements (SLA) through IoT and Blockchain, and payment for a service only transfers to the supplier when the eXergy SLA is met. The risk carrier is also tied into the network and measures the efficiency (on a scale of 1-10) against the risk. If the system is reporting low numbers on the SLA, then the compensation reflects this, and so do the risk numbers. For example, efficiency at 5 means risk at 5 and compensation at, say, 50% of the maximum billed rate via Blockchain billing under the SLA. Then risk coverage either drops by 50%, or cost of risk coverage doubles. The whole purpose of the system is to push for the highest possible quality of experience being actually delivered to the humans in the structure, and not, as we see today, to focus solely on the performance of the product or appliance in isolation.
An important project focus is eXergy (quality of work done), as the sensors and systems are measured on how well the work is done, not how well a component performs or how efficient the energy to power the appliance is. Heating, ventilation, and air conditioning (HVAC) systems are a good example of this: HVAC systems are the life support of any building, but they are rated on the mechanical performance of the system, with zero focus on the results. The stated purpose of the system is to create human thermal comfort, but that is the one thing that is not measured. AC means air conditioning, not just cooling, and comfortable air is a lot more than establishing a CO2 and temperature level threshold. Service Level Agreements mean that system performance is rated on how successfully it delivers comfort to humans, not how well an appliance performs in laboratory tests on mechanical efficiency.
Sensor networks today can use any number of network protocols or configurations, including:
- Radio-frequency identification (RFID), which uses tiny radio transponders, receivers and transmitters to automatically identify and track tags attached to objects. When triggered by a pulse from a nearby RFID reader, the tag transmits digital data back to the reader.
- Bluetooth, a wireless technology standard used to establish personal area networks (PANs) to exchange data between devices over short distances using UHF frequencies at 2.402 to 2.480 GHz as a wireless alternative to data cables.
- Power over Ethernet, or PoE, any of several IEEE standards or ad hoc systems that pass data along with electric power on a single twisted pair Ethernet cable.
- Wi-Fi (IEEE 802.11), one of the IEEE local area network (LAN) protocols for implementing wireless local area network (WLAN) communication to, from, and among computers. Wi-Fi can be implemented at various radio frequencies, such as 2.4 GHz, 5 GHz, 6 GHz, or 60 GHz.
- Wireless sensor networks (WSN), spontaneous wireless ad hoc networks that rely on one or several of a multitude of open-source or proprietary wireless connectivity protocols to wirelessly transport and cooperatively pass data from a wide variety and large number of sensors through the network to a main location.
- Low-power wide-area networks (LPWAN) or low-power wide-area (LPWA) networks or low-power networks (LPN), all names for wireless wide area networks designed to allow low bit-rate long-range communications among IoT devices such as battery-operated sensors. LPWANs may be offered by a third party as a service or infrastructure, which allows the sensor owners to deploy them in the field without investing in network infrastructure.
- Cellular 4G, or Long-Term Evolution (LTE) technology, which increases the capacity and speed of the network, but requires connection to a telecommunications carrier network.
- 5G, or fifth generation broadband cellular networks, which are just now being deployed worldwide by cellular carriers. 5G networks have greater bandwidth than any of the above, giving higher download speeds but likely much more capacity than sensors actually need.
Let’s now explore two more potential sources of complications or confusion:
- An electromagnetic (EM) field produced by accelerating electric charges the EM field propagates at the speed of light and interacts with charges and currents.
- Radio frequency (RF) is the oscillation rate of an alternating electric current or voltage or of a magnetic, electric, or electromagnetic field or mechanical system at around 20 kHz to around 300 GHz frequencies, or roughly between the upper limit of audio frequencies and the lower limit of infrared frequencies.
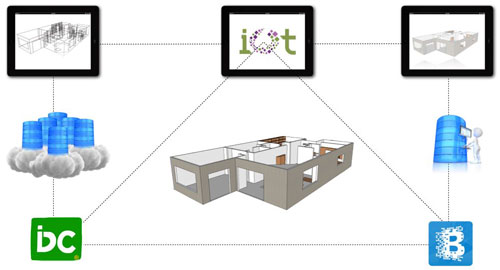
All electrical devices create both EMF and RF, and very little research has been done on the broad spectrum (pun intended) impact of those emissions. As sensors and devices proliferate from 10s to 1000s, far more research is needed. Without a good understanding of the aggregate impact of this proliferation, the eXergy rate and how it affects the end user quality measurement is affected, and the risk counter goes up. Alternating current uses more energy than Intelligent DC or even normal DC, and so the EMF/RF emissions are more powerful.
None of this is addressed by International Building Code (IBC) International Mechanical Code (IMC) or International Electrical Code (IEC), even with the number and types of devices already in use today. Many contractors, even those supposedly specialized in related fields such as environmental controls or indoor air quality, do not understand the problem, have not explored best practices around sensor use and installation, and certainly can’t point to proven best practice solutions to apply. This can lead to total project failure.
Let’s look at an issue in MetaHome to illustrate what I just said—a very simple one with big implications: water. Without quality water, the project fails, period. Currently, the City of Boulder water distribution system focuses on cross-city water quality on the outside of the meter. But inside MetaHome, we are targeting very different standards. MetaHome is focused on zone-by-zone end-user functionality. Michelle Wind (head of water quality at the City of Boulder) has sent us great information on how the City looks at water quality, but in many ways that’s at cross purposes with our end-user focus. This is important for all the sensors and systems in the project, because there is voluminous data on water and great knowledge on what end users need. Water quality is determined by its source, how it is treated, and the materials used to transport it to its destination. Water movement is a form of energy; as water moves, the quality of water delivered at the point of end use is impacted by the different stresses experienced by each component of the system and the system as a whole. This describes the eXergy of water.
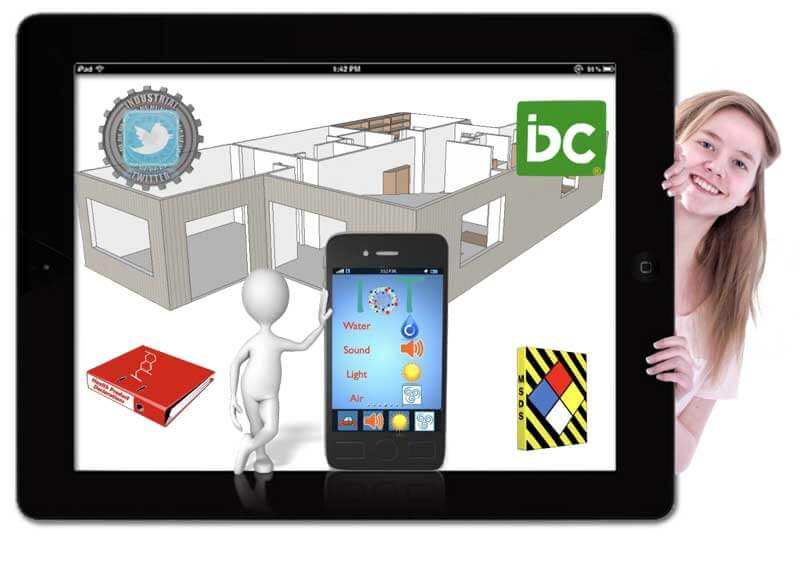
MetaHome is developing a real time Material Safety Data Sheet / Safety Data Sheet / Health Product Declaration (MSDS/SDS/HPD) system. All the interior components of MetaHome are being modeled, as there are many disparate sources of information regarding what effects different materials have on dynamic systems such as water, and vice versa. Sadly, our understanding of the safety impact of water running through different materials changes over the ages, as recent events in Michigan and the whole Kitec issue show. As we add more and more materials derived from petrochemicals and other substances (the potentially toxicity of many of which is completely unknown) to the infrastructure carrying water, let’s not be surprised at the potential catastrophes that ensue.
We started conducting due diligence by validating water sensors already embedded in appliances used in the system. In the first case, we explored the water filter on a GE Refrigerator PYE22KSKHSS, which has RFID interrogation on the primary filter. At first, the manufacturer informed us the filter sensor relayed warning messages related to the flow of water through the carbon filter. When we more closely questioned how that was done with an RFID sensor, we found out that the filter is just supposed to time out after 6 months. This is interesting, because it’s not really related to the quality of the water (we are still in correspondence with GE about this).
For example, if you did not use the fridge water dispenser, and therefore had no flow through the filter for 6 months, it would still request a new filter six months after installation. If you have reason to believe you have bad quality water entering the line and are relying on the filter to do something, it would still not request a new filter until six months after installation.This raises the question—still undecided—of whether an RFID-enabled filter is intended to improve water quality or simply sell more stuff. City water is at 40psi but the filter manufacturer states that anything above 10psi negates the function of the filters, a dilemma indeed for quality water.
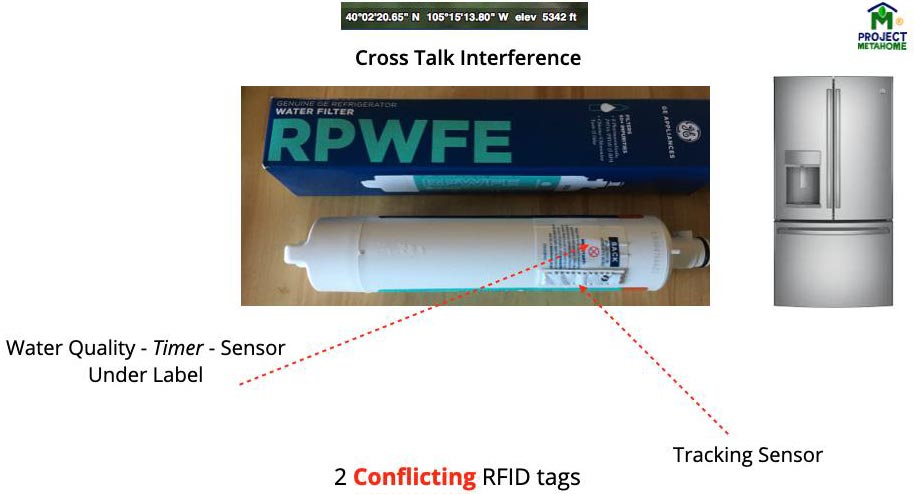
But the GE technical data calls it a water filter, so let’s proceed and treat it as such. The manufacturer stated that a filtration system is necessary in Boulder due to water quality. We then asked if the bypass system could be used to test water quality with and without a filter by taking samples with and without a filter. They have not yet responded.
One thing we noticed was that change filter warnings on the electronic panel came up after 20–30 days, not 6 months or ±180 days, which translated to around $50 ✕ 12 = $600 worth of filters. As we communicated with GE on the problem, at first they blamed Boulder City water, which we relayed to Michelle Wind. But as the onsite tech service guy explained how it worked, we realized that this is not possible. More examination discovered that a second, inventory-tracking RFID chip had been placed right alongside the primary sensor, and what was happening was co-channel Interference, which caused the master controller to register a fault. Since the only reporting options it has are “Filter OK” or “Filter Change Needed,” a malfunction causes it to default to “Filter Change Needed,” which the human end user interprets as “the filter must be bad, thus the water quality must be bad.” Was this intentional by the manufacturer to sell more filters, or a problem in QA? We are still investigating.
Sadly, the more we look at quality measurement using IoT, the less we see any meaningful relationship between what the sensor says and what the experienced quality of anything really is. The negative correlation is magnified because fewer than 1% of the devices are actually designed to measure the quality of the service being provided to the human occupants (eXergy)—which contradicts what smart homes and IoT are marketed as doing. This has been put in clear focus with air issues in 2020.
Why is this important? For adherence to quality standards to be validated, the systems have to be trusted, and for the systems to be trusted, we must understand three things: what message is conveyed by the IoT device signal, what is the real meaning of the message, and what happens to the signal and its message under adverse conditions, such as co-channel interference. Above all that is the risk calculation: if the device being reported on is a pacemaker keeping someone alive the clarity of its status message and the robustness and resilience of its signal have a higher priority than if the device being reported on is a domestic thermostat.
As we move into true smart homes with thousands of signals being transmitted every second, sorting this out will become a major priority, especially with the added complexity of smart intelligent Direct Current being added as a carrier.
This is also sadly creating pseudo-solution markets that have no relevance to the end eXergy product. As an example, when we began scrutinizing the filtered water output, multiple companies recommended multi-thousand dollar “solutions” because “No municipal system, including Boulder water, can be trusted, so you need to build your own.” Proposals stretched from whole house filter and monitoring systems to whole house plumbing and systems retrofit, but none of them could quantify how the “solution” would be better than what is in place now (which none of them had assessed). In other words, quality is poorly defined and monitored, so the concept can be misused as a marketing ploy, as we see today with air purification.
One of the most interesting parts of this project is asking experts at the supply source of a product what the sensor does. As an example, let’s look at water again. Marketing Sales and Customer Relations at GE and multiple water filter vendors of all types, both appliance and in-water-line systems, wax rhetorical about the quality of water derived from their product, but—and this is where it gets interesting—when you request (as we did with GE) a technical explanation of how it works, the rhetoric falls flat.
Basically there is only one way to define water quality for an eXergy (or really any) purpose:
a) Understand the water source
b) Understand the material the water passes through
c) Identify the quality needed at the end user point
This a – b – c method can also be used to define other SLA targets. Only when those three factors are clearly known can you set a quality standard that can be tied to an SLA.
The sensors on GE Refrigerator filters, although called water quality sensors, can only tell you the time that has passed from installation of the filter—and as we saw they even get that wrong when paired with another sensor for a different purpose. This also applies to major household brands of water filters.
One thing we started to look at early in MetaHome is sensor accuracy. If sensors are not accurate, then neither is your data, and if your data is corrupt “garbage in=garbage out” (GIGO) prevails and nothing makes sense, nor can you make action decisions based on the bad data. The dilemma is: how do you validate any sensor for accuracy? The most common response from all manufacturers is that their sensors are accurate, end of story. Then you show them 2-10 identical sensors with different readings, and the “accurate” statement changes to “accurate within degrees”—5, 10, 20 and higher percent, depending on the environment, depending on QA, etc., etc.
Cost isn’t really a factor, either. We have found that more expensive sensors can be as inaccurate as cheap ones, with the prime difference being that more expensive systems claim to self-calibrate. Of course, this again opens up another can of worms: how does it (the sensor) do that, what baseline does it use, and how does the end user validate that it has calibrated accurately? About 75% of the sensors tested have failures at point of install, and so far the most reliable way to get real data has been to use a ridiculous number of sensors to measure the same thing and report over LPWAN, then average the readings. Much slower, but far more reliable. The same issues affected the project air sensors like Foobot that have an average life of 9 months with software glitches every 60 days.
So when you see a product with a label stating “improved quality” with an attached sensor, you might want to ask exactly what the sensor is detecting (and how), as it’s probably not the quality it claims to be intended to detect.